1.Insubstantial Object Detection
Recently, the emergence of deep learning based approaches has witnessed significant advancements of object detection. Nevertheless, they still face intractable problems on some insubstantial objects captured by multispectral cameras under specific wavelength, e.g. smoke, steam and gas leak. Due to frequent occurrences of smoke poisoning, fire accident, toxic gas leakage and explosion, it is urgent and crucial to realize real-time intelligent monitoring as well as early warning for insubstantial objects. This research topic is fresh and challenging, as insubstantial objects are quite different from conventional objects from several aspects:
(1) indistinct boundary and amorphous shape;
(2) the similarity to the background surroundings;
(3) absence of color information and saliency;
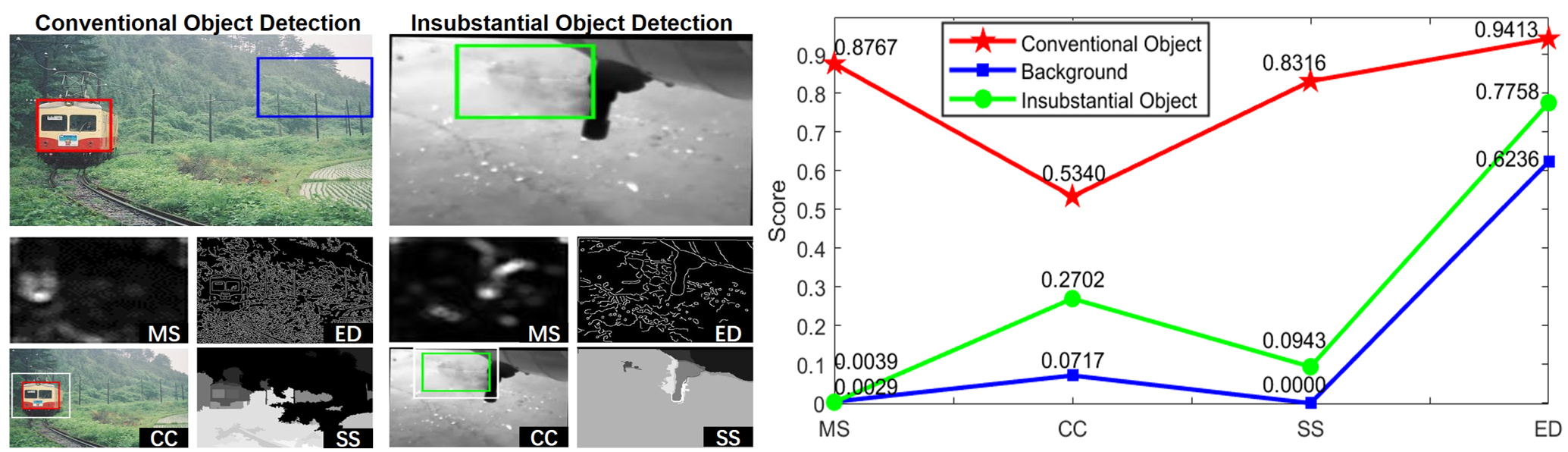
In consideration of the image cues (MS, CC, SS, ED) in classical paper what is an object, the insubstantial object is more similar to the background rather than the foreground.
2.Overall Pipeline
Consequently, the collaborative representation of spatial and temporal features is crucial under the limitation of spatial information within a static frame. In this paper, we explore a spatio-temporal aggregation framework from two aspects: First, representative spatio-temporal backbones of action recognition are introduce into our framework to evaluate their accuracy on IOD task. Second, spatio-temporal aggregation loss (STAloss) is designed to impose constraints in the three-dimensional space.
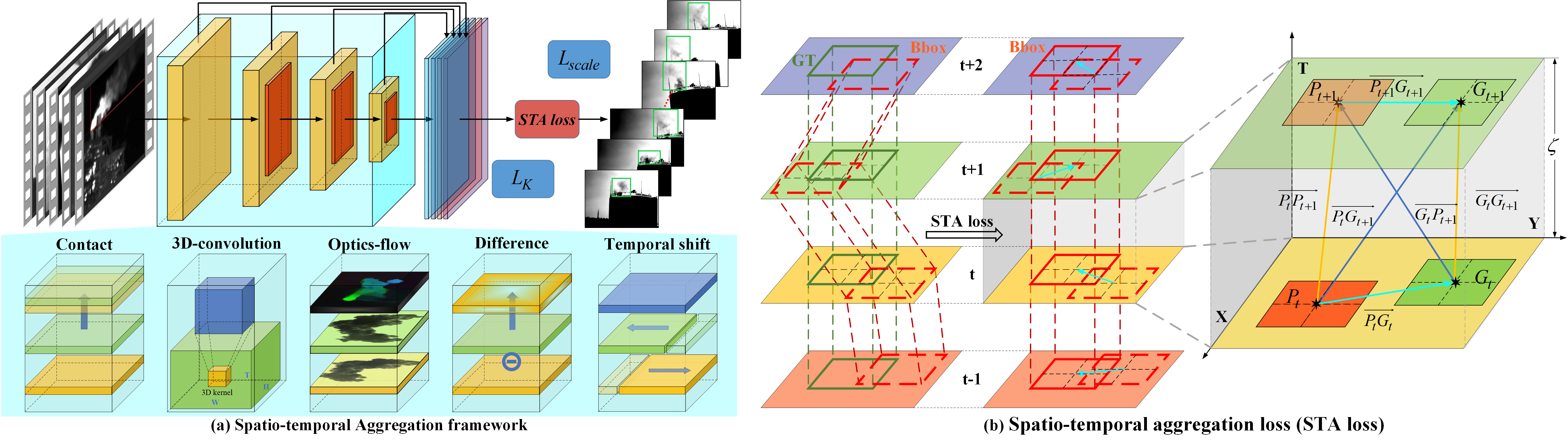
3.Experiment Results
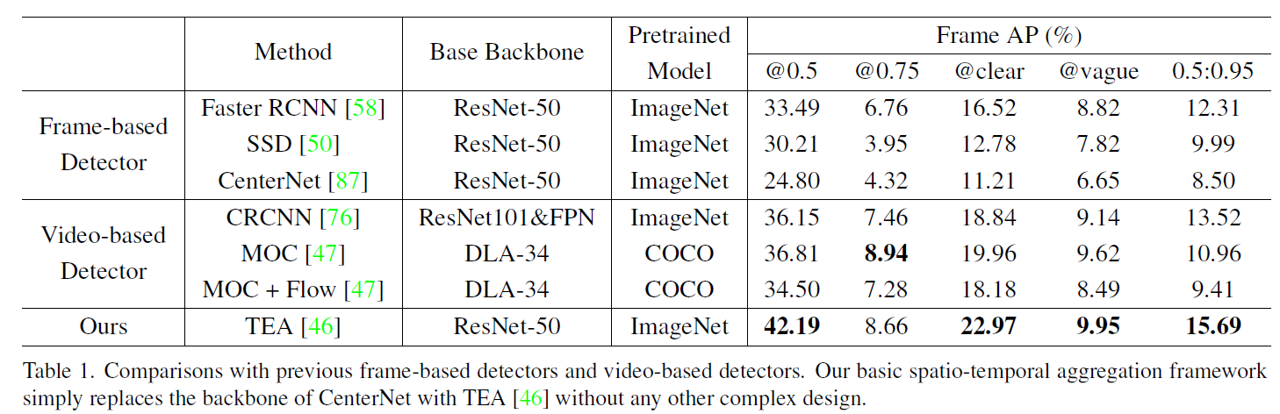
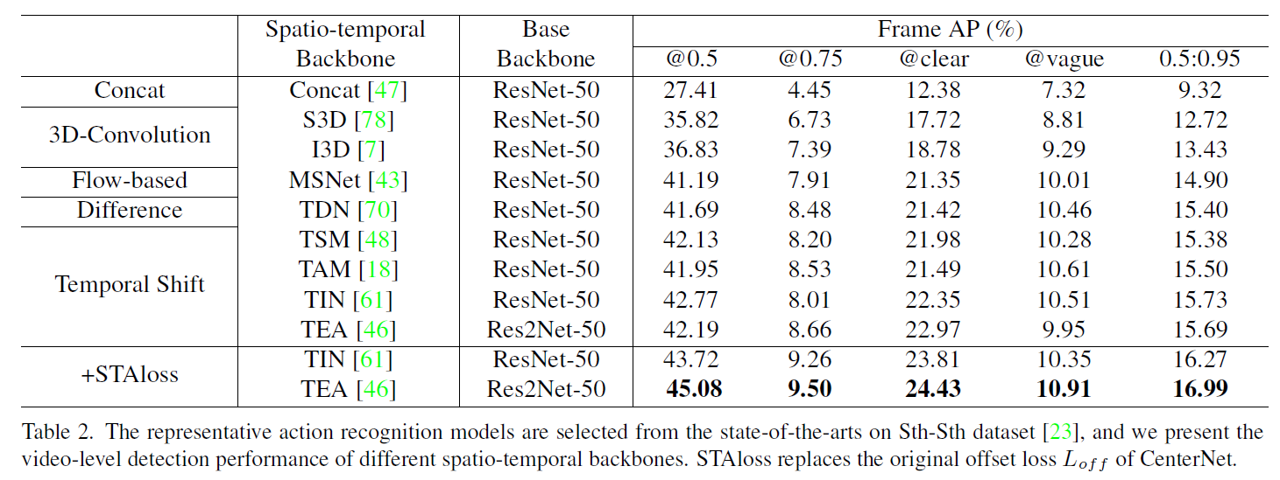
Experimental results reveal that temporal shift models have the best video-level detection performance which may preserve the feature-level integrity of spatial dimension, and STAloss can further improve the performance.